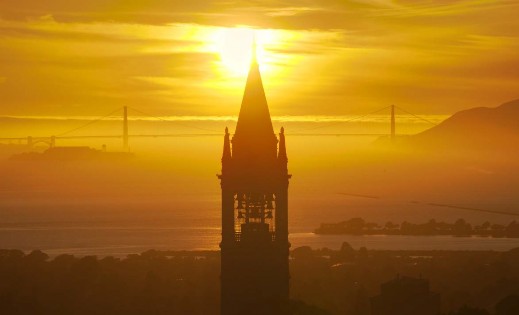
Photo by D.H. Parks. Published with author's permission. CC BY-NC 2.0
Theme: “Algorithms are Opaque and Unfair. Now What?”
Date: Friday, June 15, 2018
Location: South Hall, School of Information, UC Berkeley
Scholars, watchdogs, journalists, and industry researchers have shown that algorithms and algorithmic systems can be susceptible to claims of unfairness, bias, and opacity, among other things. Where do we go from here?
On June 15, 2018, the Algorithmic Fairness and Opacity Working Group (AFOG) held a summer workshop with the theme, “Algorithms are Opaque and Unfair: Now What?” The event was organized by Berkeley I School Professors (and AFOG co-directors) Jenna Burrell and Deirdre Mulligan and AFOG postdoc Daniel Kluttz, and Allison Woodruff and Jen Gennai from Google. The workshop was generously co-sponsored by the UC Berkeley School of Information and Google Trust and Safety.
Our interdisciplinary group of 40 participants came from a diverse set of universities and organizations, including Amnesty International, the Electronic Frontier Foundation, Facebook Research, Google, the MITRE Corporation, New America, Sciences Po, Stanford University, Uber, UC Berkeley, UC San Diego, the University of Illinois at Urbana-Champaign, and the Wikimedia Foundation.
Please read our Report of the AFOG Summer 2018 Workshop, which includes an overview and general themes that emerged from the event as well as detailed write-ups for each panel. These write-ups synthesize and extend panel discussions in ways we hope will stimulate future research and spur collaboration among stakeholders with diverse interests and backgrounds. We intend for these documents to inform an audience of researchers, implementers, practitioners, and policy-makers.
Please read our summary of the workshop here
Pre-workshop agenda
(1) What a technical ‘fix’ for fairness can and can’t accomplish. Drawing from various formal definitions of fairness (see Narayanan 2018; Kleinberg et al. 2017), researchers have recently identified a range of techniques for addressing algorithmic bias and discrimination. Most of this work frames the issue as a technical problem. This panel will discuss the types of problems that can be identified and remediated with technical solutions. In addition to discussing the varieties of technical fixes, other questions the panel will address include: What problems are beyond technical resolution? For example, could a sociologically and historically informed view of race ever be accounted for in a fairness algorithm? What other ways of acting on discrimination and bias (if not via technical fixes) are in scope? How do we identify when to partner with or hand off problems to other organizations or draw from other areas of expertise?
Panelists
Lena Z. Gunn, Electronic Frontier Foundation
Moritz Hardt, UC Berkeley
Abigail Jacobs, UC Berkeley
Andy Schou, Google
Moderator: Sarah Brown, UC Berkeley
(2) Automated decision-making is imperfect, but it’s arguably an improvement over biased human decision-making. This panel will debate the idea that because automated, decision-making tools realize greater (predictive) accuracy, they are preferable to and could even replace a human decision-maker. Sometimes this stems from the assumption that human and machine processes as cognitively similar. However, if human and machines “make decisions” that are fundamentally and qualitatively different, how do we compare and account for those differences? What metrics may apply? Cowgill and others propose counterfactual fairness as a way to investigate these questions (Cowgill and Tucker 2017; Kusner et al 2017). Furthermore, in practice, decision support tools are often positioned not to replace human roles, but to augment their decision making. In some cases, machine decision making is available but ignored or manipulated by humans to produce desired results (Christin 2017).This panel will also provide an opportunity to talk about contestability or the ways that professionals with deep expertise could engage with algorithmic decision support without delegating decision making entirely to machines.
Panelists
Angele Christin, Stanford University
Marion Fourcade, UC Berkeley
Joshua Kroll, UC Berkeley
M. Mitchell, Google
Moderator: Deirdre Mulligan, UC Berkeley
(3) Tools for user autonomy and empowerment. There is a justified concern that the rise of algorithmic decision making means a loss of human autonomy, and that this loss may cost vulnerable groups most severely (Eubanks 2018). In the push toward automated classification and decision making, how do we preserve the autonomy of people who use or are subject to these systems? What tools are available that help users to better understand, give feedback, or launch appeals against how they have been classified? Recent incidents have been exposed using general purpose public platforms: by tweeting (e.g., http://bit.ly/1dvA361), writing a Medium article (e.g., http://bit.ly/2hkGReR) or through whistle-blowing. What role does UX design play in user empowerment? What role could it play? How do we handle the way user feedback tools are manipulated by coordinated groups in ways that undermine the intent of these tools (see Tufekci 2017)? How do users organize themselves to implement new & desired features using APIs (Geiger 2016)? How do we enhance autonomy for people who are classified by these tools, but who do not interact with them directly?
Panelists
Stuart Geiger, UC Berkeley
Jen Gennai, Google
Niloufar Salehi, Stanford University
Moderator: Jenna Burrell, UC Berkeley
(4) Auditing algorithms (from within and from without). Sandvig et al (2014) define an algorithmic “audit” as a systematic process, such as a structured field experiment, for investigating and uncovering discrimination or other harms in an algorithmic routine. There is a growing subfield of research into algorithmic auditing (see, e.g., https://bit.ly/2ILPAiM). Could corporate practices of self-auditing be adapted to address fairness preemptively? How could this be made part of design methodology? What other competing or complementary practices–such as scorecards, industry standards and BKMs, or ideas from information security (such as bug bounties)–could create internal or external pressure to address and improve fairness within firms and industry-wide? What is the ecosystem of third parties that can be brought to bear on this issue? What role can journalists, academics, regulators, and users play in identifying problems and pushing for change? How can their role be best supported.
Panelists
Chuck Howell, MITRE
Danie Theron, Google
Michael Tschantz, UC Berkeley
Moderator: Allison Woodruff, Google