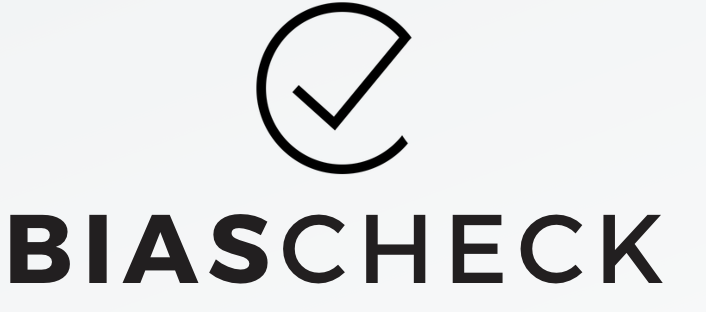
Minimizing Subjectivity in Language
Problem Statement
The problem we are focusing on is minimizing subjective biases in written language. Subconscious subjective biases are often embedded in everyday communications, even when a writer aims to be neutral. In a society that is more and more diverse and intersectional, the cultural dynamic has to adjust to prevent biases, marginalization and micro-aggressions in language. In other words, there is an imperative in our society to minimize bias, and even while many agree with this sentiment, there aren’t current tools available to automatically check one’s own biases in their writing. In the field of NLP, there aren’t any pre-existing great bias measurement tools. Existing solutions focus on toxic language or hate speech or on measuring bias in models directly. While subjective biases are more nuanced and difficult to detect, there is an opportunity to address them efficiently through fine-tuning pre-existing language models. Our product aims to bridge the gap between current solutions and the nuance of these subjective biases to help writers become more objective in their communications. As such, our primary target users are HRBPs and communication specialists seeking a bias mitigation tool. The market opportunity is in this sector is vast as current existing solutions are centered primarily around less nuanced biases, and there is a lack of user-facing products.
Market Size
The market for a bias mitigation tool in text and language models spans several growing sectors, including NLP, ethical AI, corporate DEI, and content moderation, with significant demand driven by regulatory pressures and public awareness. The NLP market alone is projected to reach $28.7 billion by 2030, and the corporate DEI market is expected to grow to $3.7 billion by 2027. The news market is estimated to be roughly $20 billion, expecting to expand at a compound rate over the next ten years. Additionally, social media content moderation, worth $10.89 billion in 2023, presents another key opportunity for such a tool. Overall, the total addressable market could be estimated at $15–30 billion by 2030, fueled by the increasing emphasis on AI fairness.
Target Customer
Primary target users are HRBPs and communication specialists and the market opportunity is vast.
Primary Use Case
BIASCheck‘s objective is to assist writing professionals in checking their subjective biases in written language. Simply, provide a text you need to check for bias. Your text first passes through a DistilBert-based classification task which classifies each sentence in your input as either Biased or Neutral along with an associated bias score. The closer the score is to 100%, the more biased your text is.
Dataset
Wiki Neutrality Corpus